Mikhail Popov
Data Science Manager & Data Scientist
I’m a polymath/generalist. I help data scientists grow in their careers and help leaders make data-informed decisions. My biggest strengths are my empathy, kindness, inclusivity, creativity, curiosity, playfulness, and my passion for learning & sharing knowledge. I believe that true success can only be achieved through togetherness.
More about me →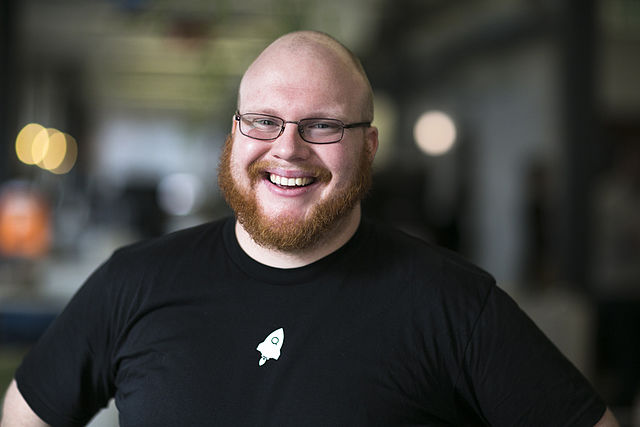
Photo by Myleen Hollero, CC BY-SA 3.0, via Wikimedia Commons